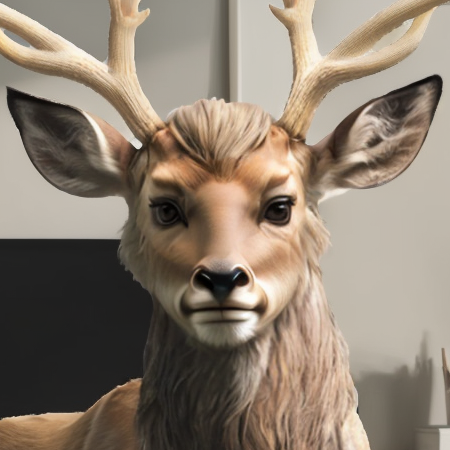
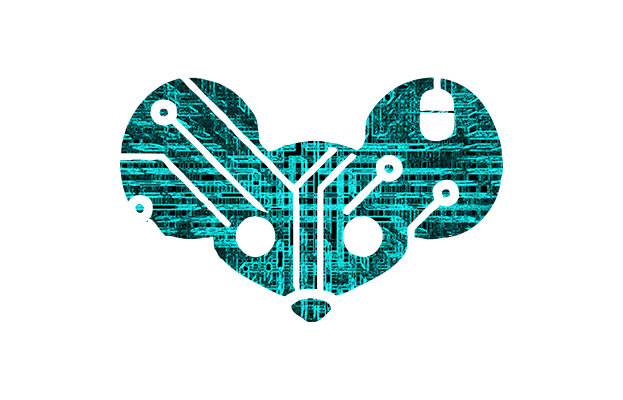
Fearing AI because of what you saw in “The Terminator” is like fearing sleeping pills because of what you saw in “Nightmare on Elm Street.”
Basically a deer with a human face. Despite probably being some sort of magical nature spirit, his interests are primarily in technology and politics and science fiction.
Spent many years on Reddit and then some time on kbin.social.
Fearing AI because of what you saw in “The Terminator” is like fearing sleeping pills because of what you saw in “Nightmare on Elm Street.”
There are people who want AI, crypto, and IoT things. If there weren’t then there’d be no money to be made in selling it.
There have been many systems developed over the years for handling decentralized data storage, decentralized user identities, and decentralized decision-making. There are excellent options out there for all this stuff.
IMO the problem is that there’s a huge “not invented here” problem, combined with a popular “ew, I don’t want to be associated with that technology (or more accurately with the group behind that technology)” reflex that has nothing to do with the technology itself. So projects like the Fediverse keep reinventing the wheel over and over, and whenever a project manages to do something right it’s rare for the other projects to abandon their own implementations to borrow from the best.
Those jobs are also being replaced by AI. Modern AIs are trained on synthetic data, which is data that was generated from source material specifically for training purposes by other AIs. AIs reformat, rewrite, and vet the source material more reliably and efficiently than humans.
AI models don’t actually contain the text they were trained on, except in very rare circumstances when they’ve been overfit on a particular text (this is considered an error in training and much work has been put into coming up with ways to prevent it. It usually happens when a great many identical copies of the same data appears in the training set). An AI model is far too small for it, there’s no way that data can be compressed that much.
Those sadly will also be replaced over time with machines and there is something we will really lose.
Not all of the things we lose will be sad, though. An AI researcher has the potential to be more thorough and less biased when it comes to digging up and interpreting resources.
In my experience the vast majority of posts about Elon Musk are from people who hate him and are tired of hearing about him.
It’s unfortunate that there’s such a powerful knee-jerk prejudice against blockchain technology these days that perfectly good solutions are sitting right there in front of us but can’t be used because they have an association with the dreaded scarlet letters “NFT.”
Not only can AI do that, it probably does it far better than a human would.
I like XKCD’s solution. Aside from the fact that it would heavily reinforce whatever bubble each community lived in, of course.
There is a certain amount of irony when people respond to a comment that mentions AI with a reflexive “AI is just a fancy autocomplete!” Without any relevance to the larger context.
Yeah. A lot of people loudly declaring that they’re switching to Linux, followed by them staying with Windows anyway.
But it’s yet another opportunity to post a comment about how much we hate cybertrucks and the people who own them, so up it goes!
Yeah, the whole concept of “national” TLDs is proving to be a rather poor one in practice. Very few of them actually make sense in the way they’re used.
Heh. I fell off of contributing in recent years, but there was a time back in the day when my edit count was in the top hundred or so. Your impression is completely wrong.
Anyway, this discussion here isn’t going to affect what the people on Wikipedia are doing, so it doesn’t really matter. I linked to the project page above and it’s quite clear that even this “AI Cleanup” project is not in any way fundamentally opposed to using AI, they’re just focused on ensuring that editors using it are adhering to Wikipedia’s guidelines. If you think AI can’t do that then clearly your concept of how AI is useful is too limited.
You’re probably assuming that someone would just go to an LLM and say “write a Wikipedia article about subject X”? That wouldn’t work well, but that’s very far from the only way to use LLMs for Wikipedia work.
For starters, it doesn’t have to actually write content at all. You could paste an existing article into an LLM and ask it “What facts in this article lack references to back them up? Are there any weasel-worded statements, or statements that don’t appear to follow a neutral point of view?” And get lists of things that require attention.
Or you could paste a poorly-worded article in and tell it to rewrite it with all the same information but better phrasing or structure. You could put a bunch of research materials you’ve gathered into the LLM’s context and tell it to write a summary in the style of a Wikipedia article, with references to the sources for each fact mentioned. Obviously you’d check the LLM’s work afterward and probably do some manual editing, but this would be a great time and effort saver to get a first draft written. You could take an existing article and tell the LLM that some particular fact had changed or been discovered to be incorrect and ask it to rewrite the relevant parts to account for that.
Wikipedia is in many, many languages. You could have a multilingual LLM automatically compare the contents of different language versions of a Wikipedia article and ask it to spot differences in content or tone. You could have an LLM translate an article from one language to another as a starting point for creating an article in that new language.
You could have the LLM check the references of an existing article - look up each referenced work on the web and see whether it genuinely says what the article that’s using it as a reference says. It could flag all manner of subtle problems that way. Perhaps the reference sounds biased, or whoever used it as a reference misinterpreted it, or the link was simply incorrect and points to unrelated material. Being able to have an AI do a first-pass check of all that in a completely automated way would save huge amounts of time.
This is all just brainstorming off the top of my head, so I’m sure there’s plenty of other good uses that aren’t coming to mind.
The purpose of this project is not to restrict or ban the use of AI in articles, but to verify that its output is acceptable and constructive, and to fix or remove it otherwise.
There’s nothing fundamentally wrong with LLMs. Users just need to know their capabilities and limitations and use them correctly. Just like any other tool.
They’re not talking about the same thing.
Last week, researchers at the Allen Institute for Artificial Intelligence (Ai2) released a new family of open-source multimodal models competitive with state-of-the-art models like OpenAI’s GPT-4o—but an order of magnitude smaller.
That’s in reference to the size of the model itself.
They then compiled a more focused, higher quality dataset of around 700,000 images and 1.3 million captions to train new models with visual capabilities. That may sound like a lot, but it’s on the order of 1,000 times less data than what’s used in proprietary multimodal models.
That’s in reference to the size of the training data that was used to train the model.
Minimizing both of those things is useful, but for different reasons. Smaller training sets make the model cheaper to train, and a smaller model makes the model cheaper to run.
If I could get glasses that told me “that guy enthusiastically greeting you by name right now is Marty, you last met him in university in such-and-such class eight years ago” I would pay any amount of money for that.
“Doxing people” and “recognizing people” have a pretty blurry border.
And yet it’s accomplishing those tasks. I guess that means “understanding” wasn’t necessary for them after all.
Entertainment.
If you think it’s supposed to be predictive you’re perhaps confusing it with futureology, which is a more scientific field.