
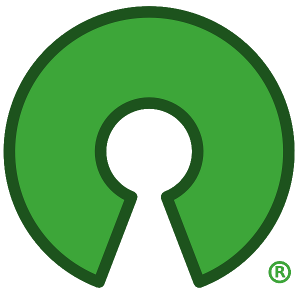
I’ve been tempted to ditch my current password manager and move to bitwarden. I think this is the final push I needed.
I’ve been tempted to ditch my current password manager and move to bitwarden. I think this is the final push I needed.
Imagine how good it will feel to let them waste all their money and lose! Go make it happen. VOTE!
Similar to previous reply about MATE with font size changes, I do that with plasma. I hadn’t seen plasma big screen you linked, I’ll definitely try that one out. I’ve wondered about https://en.m.wikipedia.org/wiki/Plasma_Mobile? Like these sort of niche projects don’t always get a lot of attention, if the bigscreen project doesn’t work out, I’d bet the plasma mobile project is fairly active and given the way it scales for displays might work really well on a tv
Speaking of scaling since you mentioned it. I have noticed scaling in general feels a lot better in Wayland. If you’d only tried it in X11 before, might want to see if Wayland works better for you.
First a caveat/warning - you’ll need a beefy GPU to run larger models, there are some smaller models that perform pretty well.
Adding a medium amount of extra information for you or anyone else that might want to get into running models locally
If you look at https://ollama.com/library?sort=featured you can see models
Model size is measured by parameter count. Generally higher parameter models are better (more “smart”, more accurate) but it’s very challenging/slow to run anything over 25b parameters on consumer GPUs. I tend to find 8-13b parameter models are a sort of sweet spot, the 1-4b parameter models are meant more for really low power devices, they’ll give you OK results for simple requests and summarizing, but they’re not going to wow you.
If you look at the ‘tags’ for the models listed below, you’ll see things like 8b-instruct-q8_0
or 8b-instruct-q4_0
. The q part refers to quantization, or shrinking/compressing a model and the number after that is roughly how aggressively it was compressed. Note the size of each tag and how the size reduces as the quantization gets more aggressive (smaller numbers). You can roughly think of this size number as “how much video ram do I need to run this model”. For me, I try to aim for q8 models, fp16 if they can run in my GPU. I wouldn’t try to use anything below q4 quantization, there seems to be a lot of quality loss below q4. Models can run partially or even fully on a CPU but that’s much slower. Ollama doesn’t yet support these new NPUs found in new laptops/processors, but work is happening there.
It’s a good thing that real open source models are getting good enough to compete with or exceed OpenAI.
It has been on my list to figure out how to move to forgejo, need to do it soon before the migration process breaks or gets awful.
Coming from c# then typescript and nextjs, rye feels very intuitive and like a nice bridge / gateway drug into python.
VS Code’s git features are pretty good for staging changes, resolving merge conflicts, pushing changes. I still do most branch changing and creating with the CLI, and yeah, any sort of problem generally needs the CLI.
We’ve also been using graphite at work and there’s a lot I like about graphite. They have a VS Code extension I haven’t used in a while but their CLI is pretty nice
Lan-mouse looks great but keep in mind that there’s no network encryption right now. There is a GitHub ticket open and the developer seems eager to add encryption. It’s just worth understanding that all your keystrokes are going across the network unencrypted.
More than distro hopping maybe try out a zen kernel or compiling kernel yourself and changing kernel config and scheduler, or a newer version of the stock kernel?
I’m not super current on what’s in each kernel but I’d expect latest mainline to handle newer processors better than some of the older stable kernels in some of the more mainstream slower releasing distros.
Ran Asahi for several months, tried it out again recently. It’s good/fine, I just don’t love fedora.
There’s some funkiness with the more complicated install, the AI acceleration doesn’t work, no thunderbolt / docking station.
MacBooks are great hardware but I don’t think they’re the best option for Linux right now. If you’re never going to boot into macOS then I’d look for x13, new Qualcomm, isn’t there a framework arm64 option now or was that a RISC module?
I’m also assuming you’re not looking to do any gaming? Because gaming on ARM is not really a thing right now and doesn’t feel like it will be for a long while.
Really love arch and the AUR. I’ve been tempted to get nix set up for the rare cases when there’s no AUR package or the AUR package is unmaintained. I figure if there’s no package in the AUR or nixpkgs, it’s probably not worth running.
btop reports some gpu, network and disk information that I don’t think shows up in htop, feels a bit more comprehensive maybe? Both are fine, but I too use btop, it’s nice.
Random trivia: I think btop has been rewritten like 3-5 times now? It’s sort of an inside joke to the point that someone suggested another rewrite from C++ to Rust ( https://github.com/aristocratos/btop/issues/5 ). I guess the guy just likes writing system monitoring console apps.
Taking ollama for instance, either the whole model runs in vram and compute is done on the gpu, or it runs in system ram and compute is done on the cpu. Running models on CPU is horribly slow. You won’t want to do it for large models
LM studio and others allow you to run part of the model on GPU and part on CPU, splitting memory requirements but still pretty slow.
Even the smaller 7B parameter models run pretty slow in CPU and the huge models are orders of magnitude slower
So technically more system ram will let you run some larger models but you will quickly figure out you just don’t want to do it.
Respect, but…
FWIW they didn’t merge it, they closed the PR without merging, link to line that still exists on master.
The recent comments are from the announcement of the ladybird browser project which is forked from some browser code from Serenity OS, I guess people are digging into who wrote the code.
Not arguing that the new comments on the PR are good/bad or anything, just a bit of context.
DNFTA